How Linguistic Diversity Affects Speech-to-Text Accuracy
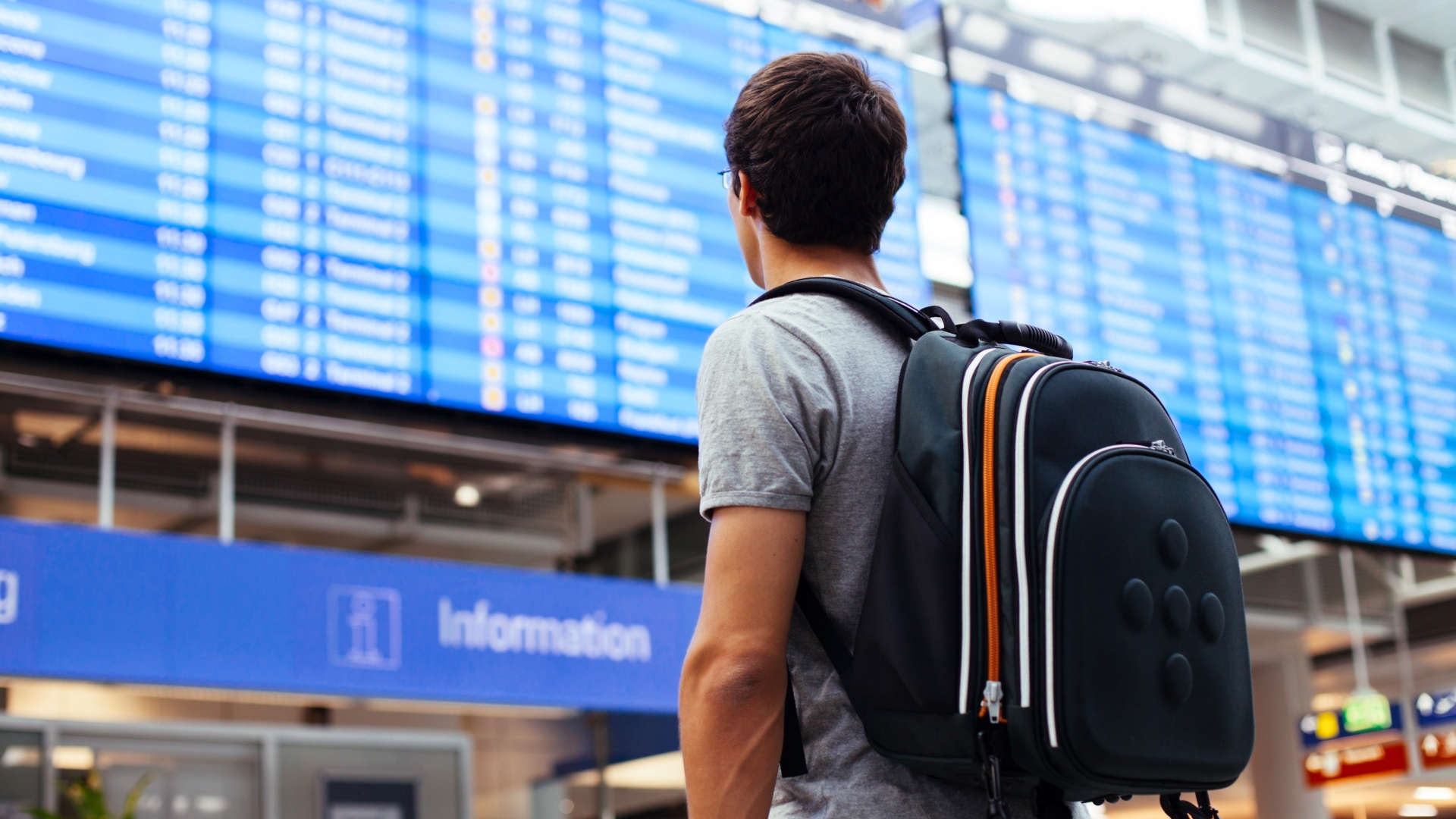
June 26, 2023
By Zuzana Ďaďová in Blog
Speech-to-text models are improving every day. As Word Accuracy differs from language to language, it often leads to a question: can a language type have an impact on the accuracy of a speech-to-text model?
In this article, we will explore language types based on their grammatical structure and how these can affect speech-to-text Word Accuracy but not necessarily the perceived accuracy (understanding) by a person.
How Is Word Accuracy Measured?
Word Accuracy is a percentage value that expresses how many words have been correctly transcribed with respect to a reference transcription.
Simply said, it is obtained by subtracting the number of word errors made by the system from the total number of words in a reference transcription (which is then converted into a percentage value):
Word Accuracy = ((Total Number of Words – Word Errors) / Total Number of Words) * 100
What is considered a word error?
- Substitutions (we have good food => we have good mood)
- Deletions (we talked about it with her => we talked it with her)
- Insertions (the friend who says that => the friend and who says that).
Users often believe that this percentage is the most important and that it is a univocal measure of the system's success. However, this is not always the case because if the Word Accuracy is 65%, the perceived accuracy (the readability of the text and the presence of the relevant information) can be much higher, especially in inflecting languages like Russian or German.
Linguistic Diversity
Although Word Accuracy depends primarily on a system’s architecture and the training data (e.g., the quantity and quality of data), another aspect influences these measures: the typological characteristics of a language.
Linguists realized almost two centuries ago that there are two types of languages in the world, more analytical languages and more synthetic languages, depending on their words’ structure complexity. To better explain how they differ, we first need to understand what a morpheme is.
Words With Many and a Few Morphemes
A morpheme is the smallest meaningful unit of language. A word can consist of one or several morphemes. Let's look at some examples:
- The word dog has only one morpheme: dog. The word dogs has two morphemes: dog and the plural morpheme s.
- The Czech word nezahodil (“he did not throw away”) contains four morphemes: ne (“not”), za (“away”), hodi (“throw”), l (past, masculine).
But let us return to the definition of the two types of languages:
- Analytic languages tend to separate morphemes, i.e., words often contain only one or two morphemes (e.g., natural science in English).
- Synthetic languages are those that accumulate more morphemes in one word (e.g., Naturwissenshaft in German).
Of course, there are languages such as French, Portuguese, Spanish, which have certain analytic features, e.g., the lack of declension of nouns and adjectives (con mi mejor amiga, para mi mejor amiga, de mi mejor amiga) and, at the same time, synthetic features, such as the conjugation of verbs (he tirado, tiré, tiré, tiraré, tirará, tiraríamos).
The Number of Words Matters
Analytic languages are characterized by a much lower number of words and a higher number of word combinations than synthetic languages.
On the other hand, synthetic languages have more words because they are morphologically richer thanks to compounds, affixes, and desinences. Let’s compare ways of saying dog in English (analytic language) and pes, its Czech equivalent (synthetic language):
English | Czech |
---|---|
A dog | pes |
I see a dog | vidím psa |
About a dog | o psovi |
With a dog | se psem |
For a dog | pro psa |
Thus, in analytic languages, every single word (dog in this case) occurs more frequently in the speech-to-text language model. In other words, each word is much more frequently represented in different contexts than in the case of synthetic languages.
So, while a linguistic model of an analytical language works with only 300 thousand frequently occurring words, that of a synthetic language works with over a million words.
Thanks to this fact, there is a tendency for higher accuracy of speech-to-text transcription in analytical language models, such as English, Danish, Chinese, Bulgarian, Vietnamese, Thai, and partially Spanish, French, or Italian.
In more synthetic languages, such as Russian, Czech, Slovak, Polish, German, Hungarian, and Turkish, the accuracy may be slightly lower due to the very strict measurements mentioned above.
Does Speech-to-Text Work Worse for Languages With More Complex Structures? Yes and No
If we look at the error rate, it is going to be higher in synthetic languages because when measuring the accuracy of a speech-to-text model, it is not the morphemes that are evaluated as correct or incorrect but the words. And given that sentence with the same meaning usually has more words in analytic languages than in synthetic, there is a higher chance of error in the latter: She did not throw it away (English) vs. Nezahodila to (Czech).
For example, the already mentioned Czech word Nezahodila means “She did not throw away”. If the word root is transcribed correctly and only the -a suffix for a female is missing (changing the meaning to “He did not throw away”), the word is still evaluated as incorrect and gets 0% accuracy, although most of its meaning stays the same.
In English (an analytical language), transcribing She did not throw away wrongly as He did not throw away will be considered 80% accurate.
Nevertheless, if we read the text (which is especially obvious for the Czech example above with a 0% Word Accuracy), these errors do not always pose an obstacle to understanding the text.
Let’s check out mistaken transcriptions – especially the one where the word's root (the most significant part of the word) is correct, but the suffix is not – and compare their Word Accuracy percentages to the actually perceived accuracy:
Reference Transcription |
Real Transcription |
Perceived Accuracy |
Word Accuracy |
---|---|---|---|
[CZ] Zhasli světlo. (They turned off the light.) |
Zhasl světlo. (He turned off the light.) |
Very high |
50% |
[TR] Yemek hakkında konuşmaya gelmedi. (She didn´t come to speak about food.) |
Balık hakkında konuşmaya gelmedi. (She didn´t come to speak about fish.) |
Quite high |
75% |
As you can see, despite the low Word Accuracy percentages, the perceived understanding is very high.
Conclusion
Apart from the quality of training data and training processes, the type of language can also influence the accuracy of a Speech-to-text model. Synthetic languages (which have more words in total) may show lower accuracy in percentage, but they are not necessarily worse in perceived accuracy.
Therefore, it is essential to keep in mind that Word Accuracy is only one way of testing the performance of speech recognition models that may indicate the model’s quality.
Apart from that, the model’s evaluation can be done in a more qualitative way, i.e., based on a user’s perception (understanding) of the transcription, given that the readability of the text and the presence of relevant information is key for the users.